"Lyrics transcription for humans": revisiting AudioShake's transcription benchmark
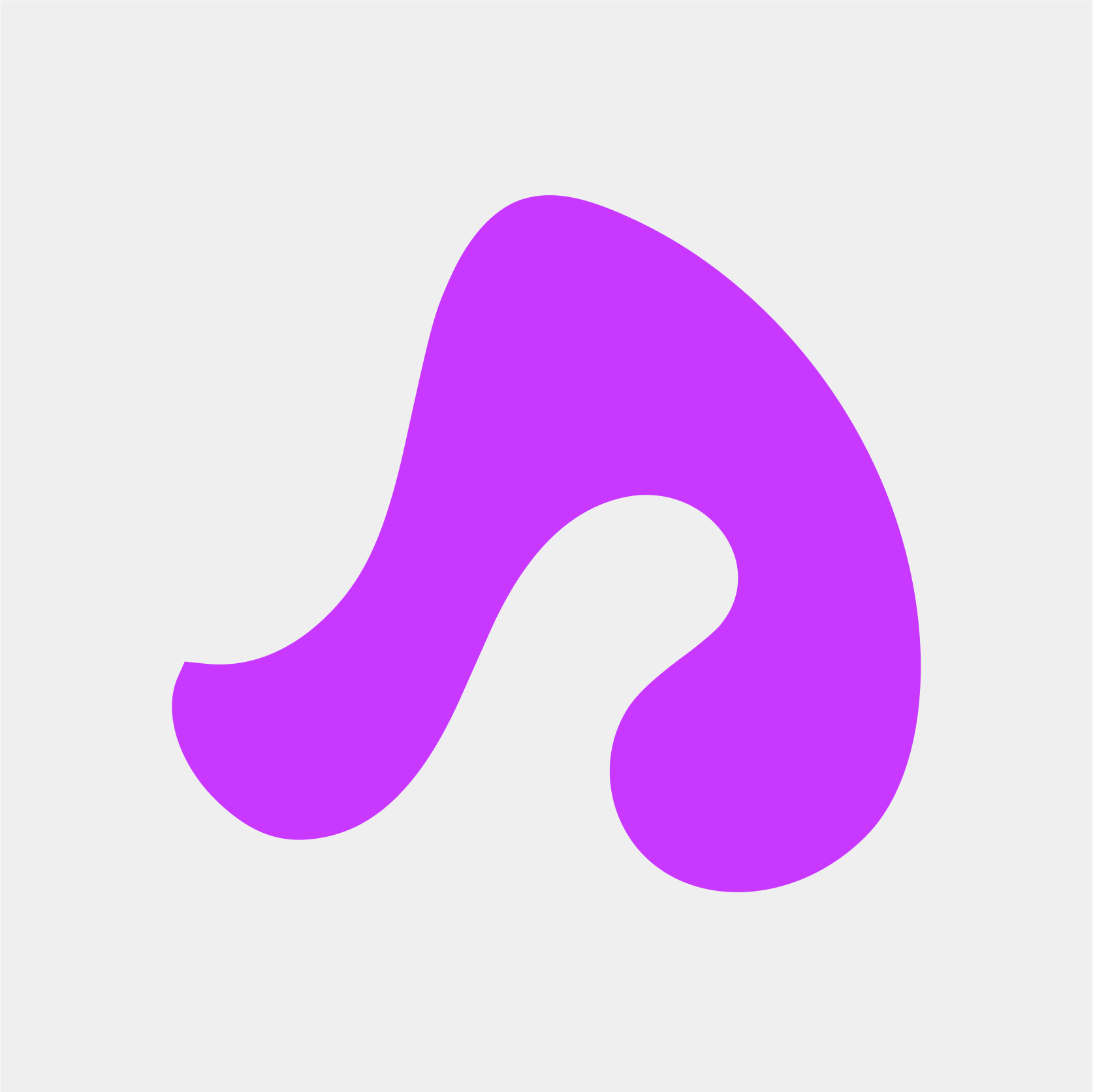
Last year, AudioShake’s research team released the Jam-ALT benchmark, comparing the accuracy of lyrics transcription systems. Their short paper showed that AudioShake’s lyric transcription service far outperformed open-source solutions, capturing lyric-specific nuances and offering higher readability.
This year, the team will present the benchmark as a full-length paper at ISMIR, the leading music information retrieval conference. The extended paper features results from a wider range of models, including AudioShake’s latest (v3) transcription model, and a more in-depth analysis of the strengths and weaknesses of different models.
Published by AudioShake Research last year, Jam-ALT is based on an existing lyrics dataset, JamendoLyrics, to make it more suitable as a lyrics transcription test set. Focusing on readability, Jam-ALT revises JamendoLyrics to follow an annotation guide that unifies the music industry’s conventions for lyrics transcription and formatting (in particular, regarding punctuation, line breaks, letter case, and non-word vocal sounds). It also fixes spelling and transcription mistakes found in the original dataset.
The paper measured performance by evaluating the word error rate (WER), as well as newly proposed punctuation- and formatting-related metrics. AudioShake’s transcription system is evaluated alongside previously published systems (Whisper, OWSM, LyricWhiz). The results shown in Table 1, showed that AudioShake’s latest system outperforms all of the above on all metrics by a large margin, with a 57% reduction in overall WER compared to Whisper v2. AudioShake also significantly improved upon its earlier transcription model (v1), reducing the error by 38 %.
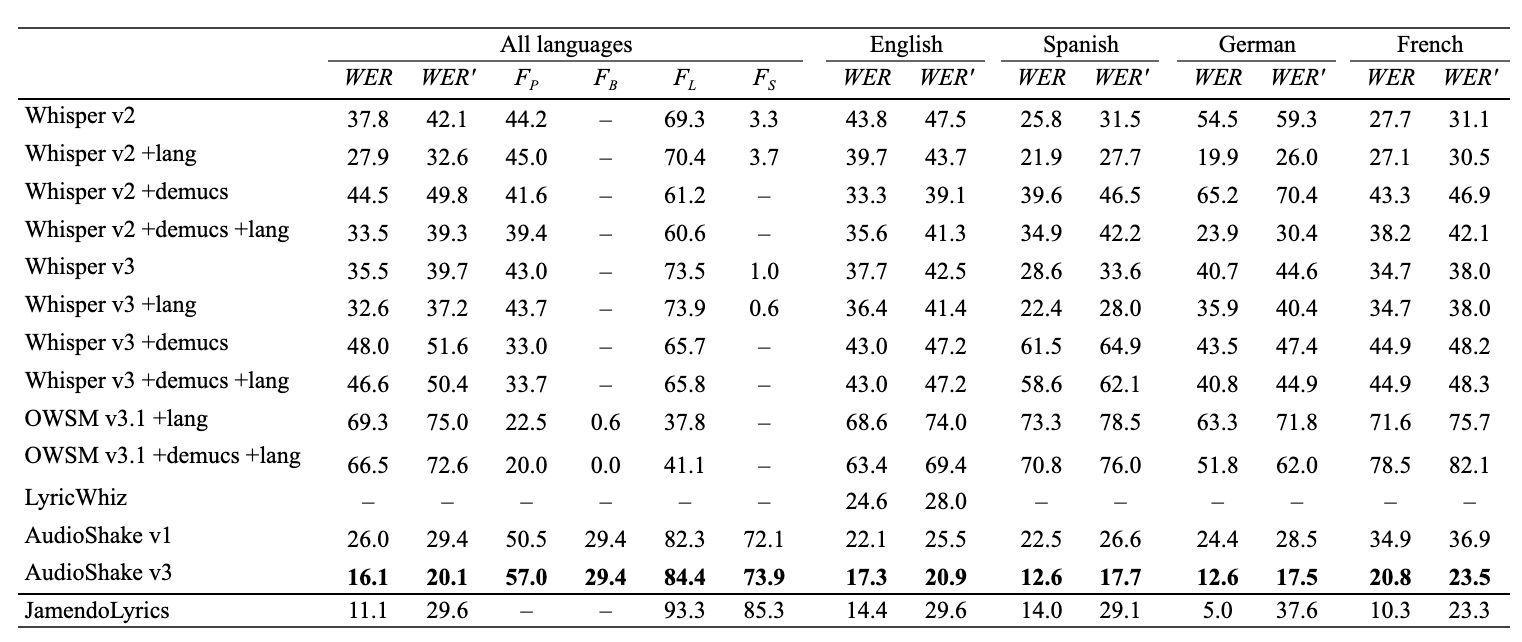
Benchmark results (all metrics shown as percentages). WER is word error rate (lower is better), WER′ is case-sensitive WER, the rest are F-measures (higher is better; P = punctuation, B = parentheses, L = line breaks, S = section breaks). +demucs indicates vocal separation using HTDemucs; +lang indicates that the language of each song was provided to the model instead of relying on auto-detection. The last row shows metrics computed between the original Jamendo Lyrics dataset as the hypotheses and our revision as the reference.
While the evaluation run by AudioShake Research demonstrated the strength of our models, it also shows the potential of these readability-aware models for lyrics transcription–specifically, how these models could facilitate novel applications extending beyond the realms of metadata extraction, lyric videos, and karaoke captions.